Table of contents
Summary
Most people agree that AI can be useful in experience research, but we’re still learning the best ways to use it. A startup called Synthetic Users is applying AI to replace discovery interviews. To test that concept, I ran a study comparing the output from Synthetic Users to interviews with real human beings. The comparison showed the current state of the art in generative AI can be useful for suggesting issues that customers might care about, but to me the AI output was misleading and incomplete when asked to take the place of interviews. I think the results suggest that AI in its current form should be used to summarize and brainstorm research, rather than replacing it.
The situation: AI is creeping into experience research
Generative AI is being applied to almost every field of business, so it’s no surprise that people are trying to use it in experience research. Most of the experiments have been around creating questionnaires and summarizing data, but a startup called Synthetic Users has gone further, completely replacing the needs discovery process with synthetic customer interviews created by AI. The company uses AI to both create the questions and create the customer responses to them, as if you had interviewed real people.
The idea is appealing because discovery interviews are painful and tedious for most companies: the traditional approach to discovery takes a lot of time and money to recruit and schedule interviews, conduct them, and then organize the results. Often companies tell me the process is so painful that they skimp on or even skip the discovery process. So the idea of dramatically compressing discovery is intriguing.
Because there are no actual human beings involved in the Synthetic Users process, it is faster and cheaper than other forms of discovery research. The benefits claimed by Synthetic Users include:
- Setup in minutes
- Synthesize results in seconds
- Ask instant followup questions
- “The AI feedback lined up with human feedback over 95% of the time.” – A user, quoted in the company home page
- Synthetic interviews are “infinitely richer” and more useful than the “bland” feedback companies get when they survey real people, according to the company’s cofounder
The shootout: Real versus AI-synthesized interviews
A growing number of studies have tested the ability of generative AI to simulate the results of opinion surveys and behavioral tests (examples here and here). But there hasn’t been as much work on AI in qualitative research. Most of the articles I could find focused on anecdotes and opinion (examples here and here), plus one sharp-edged comparison by a Berkeley professor (here). I decided to do a real-world test to gather some data. I ran the same study on Synthetic Users and on UserTesting, to compare synthesized interviews with real ones. (I also tried asking the same questions directly to ChatGPT; you’ll see those results at the end of the article.)
Full disclosure: I’m a UserTesting employee and can’t claim to be completely unbiased, but I did my best to be objective in this comparison. I also saved the raw output from all three platforms, so you can make your own comparisons. You can find links to the raw files at the end of this report. I’m very interested in your thoughts and followup questions. Feel free to dig into the data on your own.
Background on the platforms I tested
In the beta version of the Synthetic Users system, you enter a few lines of information about your target customer and product idea, and within minutes the AI makes up the users and synthesizes interviews with them. The “interviews” read as if you had done very detailed discovery interviews on the product idea. You receive a transcript of the interviews, demographics and psychographics on the synthesized users, and a summary. You can share this information in the same ways as a real interview.
UserTesting is a research platform that enables you to give questions and tasks to real people who are members of an online panel. Using their computers or smartphones, they record themselves doing tasks and answering your questions. You receive a transcript and video of their responses, plus an AI-generated summary of the results. Depending on how you configure the test, the process can take a few hours to a couple of days.
How I structured the test
I wanted to test the AI’s ability to extrapolate, by studying a product idea that’s not currently on the market, but could be easily understood and would likely generate discussion. The product idea I chose was a rideshare service that uses flying cars.
I asked for six interviews with people who have to commute to work at least three days a week, have a commute longer than an hour each way, and have at least two school-age kids at home. (Those requirements turned out to be a challenge on UserTesting, which I’ll describe below.)
Here’s how the test creation process worked in Synthetic Users. First the system asked me to describe the target customer:
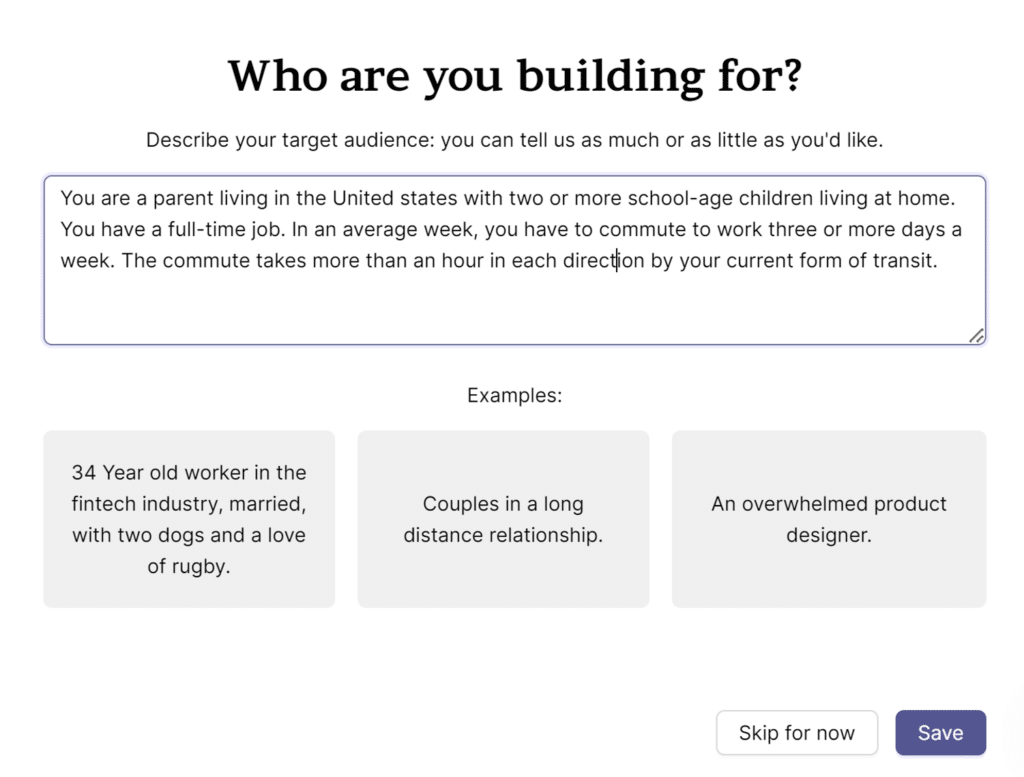
Here’s the text I entered: “You are a parent living in the United States with two school-age children living at home. You have a full-time job. In an average week, you have to commute to work three or more days a week. The commute takes more than an hour in each direction by your current form of transit.”
Synthetic Users then asked me to specify the pains and needs of those customers. This confused me a little, because one of the purposes of discovery interviews is to discover the problems of users rather than assume them. This turned out to be an important difference between Synthetic Users and real interviews, although I didn’t realize it at the time. I followed instructions and added four customer pains.
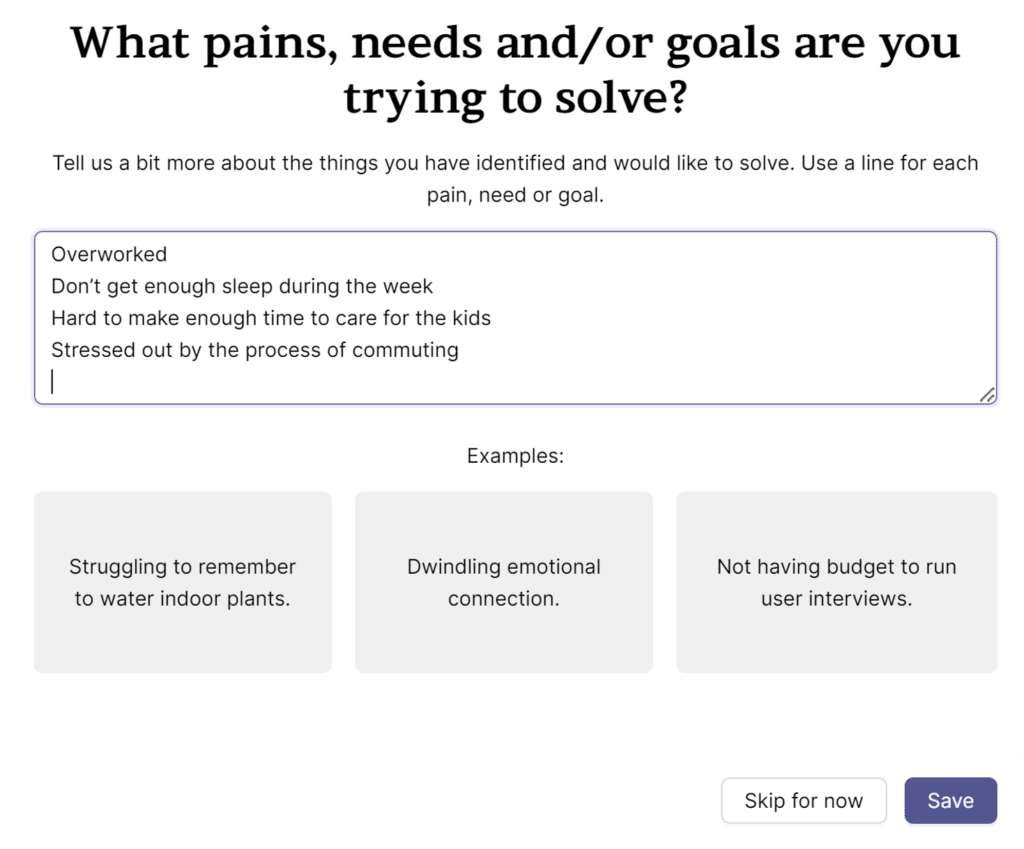
Here’s the text I used:
- Overworked
- Don’t get enough sleep during the week
- Hard to make enough time to care for the kids
- Stressed out by the process of commuting
Finally, I was asked to input my product idea:
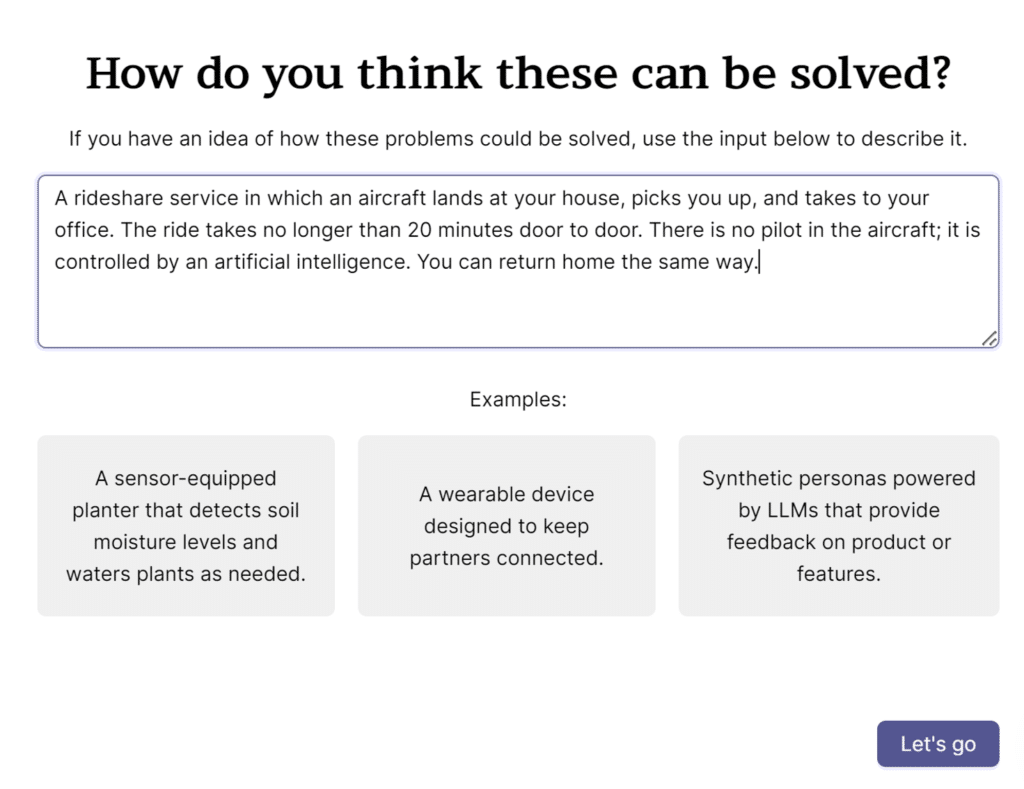
Here’s what I wrote: A rideshare service in which an aircraft lands at your house, picks you up, and takes you to your office. The rids takes no more than 20 minutes door to door. There is no pilot in the aircraft; it is controlled by an artificial intelligence. You can return home the same way.
(Yeah, there is a misspelling in there. I’m embarrassed, but it didn’t seem to confuse the AI, which in itself is interesting.)
After that, the “test” was ready to go.
As much as I could, I duplicated this process in the UserTesting study. The customer description was turned into screener questions, and the product description was included in the test plan. I asked a series of questions that matched as much as possible the topics covered by the Synthetic Users reports. And I turned the customer problems into rating scale questions so I could measure how common they are. You can see my full test plan here.
What I found: Promise and problems
As advertised, Synthetic Users was extremely fast and convenient, and the interview “transcripts” were remarkably fluent and easy to read. But the more I dug into the details, the less comfortable I was with the synthesized interviews. I didn’t get the surprises and quirkiness you get in real-world interviews, the system made mistakes, and the interviews made up by Synthetic Users sounded slick and repetitive to me. Here are the specifics:
Speed: Synthetic Users is faster. It took less than half an hour to create my test by filling in the blanks in a web form (most of the time was thinking time to be sure I got the prompts right). Once I finished entering my data, the six synthesized interviews were generated and summarized in about three minutes. The whole process took less than an hour.
In UserTesting, the process took much of the day. I chose to do self-interviews (in which you write out the questions in advance and have people read and respond to them) because that’s faster than live interviews. To run the test, I needed to write test plan questions and screener questions (a couple of hours) wait for response to arrive (a couple of hours, although I could do other work while I waited) and then analyze the results (potentially multiple hours, but that was helped by a new AI-driven summarization feature in the UT platform).
I ran into one problem with the UserTesting study, though: at first the tests were very slow to fill. I checked the responses to my screener, and found that the question about length of commute was eliminating almost all participants. Apparently there aren’t a lot of people who commute to work three or more times a week, have two school age kids, and have a commute over an hour each way. I relaxed the commute time to half an hour each way, and the test filled quickly.
This raises an interesting issue about Synthetic Users. In their system, you enter the exact demographics and problems you want people to have, and the system faithfully creates those people – even if they don’t actually exist in the real world. To verify that, I ran a separate test with Synthetic Users that asked for participants who had walked on the Moon during the Apollo landings, but were now in their 40s and working in people management roles. Synthetic Users cheerfully returned six people with those characteristics. Three of them were women (the Apollo astronauts were all men), and all of them were in their 40s – even though no one currently in their 40s had even been born yet at the time of the last Apollo mission in 1972.
This means that you can’t currently count on Synthetic Users to test the reality of the customers you’re asking for. You’ll need an external source of truth for your customer definition, one that you know is correct in all of its specifics.
In other words, you need to do research before you can use Synthetic Users to synthesize research. This is especially important for the customer problems that you enter into the Synthetic Users system – Synthetic Users is not testing those problems for you, it’s assuming they exist, and using them to synthesize the users.
Quality of transcript: Synthetic users was perfect. The transcripts from the synthetic interviews were beautifully formatted, in perfect English, very well expressed, and had no grammatical errors or mis-transcribed words. They read like professional first-person essays. You could easily share them with your company without hesitation. The UT transcripts read the way people actually speak, with many repeated words, half-completed sentences, “ums,” and the occasional mis-transcribed word. They are not as easy to read, and you’d probably want to do some cleanup before sharing them.
Which version you prefer may depend on your needs: Do you need something that reflects the speech of real people, or do you need something that’s easy to read?
Quality of video: Synthetic Users did not participate. UserTesting delivers videos of the people taking the test. In this case, I turned on face recording so you could see the participants and judge their expressions. Since there aren’t any real people being interviewed by Synthetic Users, there is no video to watch, or video clips to share.
In the absence of videos, it’s hard to get the sort of empathy and intuitive understanding of customers that you get from real interviews. To get a feel for what that’s like, here’s a short highlight reel from my test, in which people discuss the problems with their current commutes:
Quality of responses: Synthetic Users feels repetitive and sometimes artificial. Synthetic Users gave me what looked like six different interviews, but the “users” all sounded very similar to one another. Many of them raised the same issues with almost the same phrasing. For example, here are quotes from five different Synthetic Users interviews about overwork and lack of sleep:
Synthetic Users excerpts on overwork and sleep
- “I also try to establish a healthy work-life balance by setting boundaries and making time for self-care. In terms of sleep, I have experimented with different sleep schedules and tried to create a calm and conducive sleep environment.”
- “To combat being overworked, I have tried to prioritize self-care by setting boundaries and allocating time for rest and relaxation. This includes trying to maintain a consistent sleep schedule and engaging in activities that help me unwind.”
- “To cope with my overwork and lack of sleep, I have tried to prioritize my tasks and manage my time more efficiently. I have also attempted to establish a consistent sleep schedule and create a calming bedtime routine to improve my sleep quality.”
- “To manage being overworked, I prioritize my tasks, delegate when possible, and practice time management techniques. For the lack of sleep, I try to establish a consistent bedtime routine and make sleep a priority.”
- “To manage being overworked, I have tried to prioritize tasks and delegate when possible. To improve sleep, I have established a bedtime routine and tried relaxation techniques.”
It is very unusual to get that sort of unanimity and repeated phrases in a test of real users. If you found this sort of repetition in real interviews, it would indicate one of those rare situations where there was a deep consensus across society.
In contrast, the UserTesting responses were more diverse in both content and wording. They were also less grammatically correct. Here are the raw, uncorrected transcripts from when I asked real people if they get enough sleep at night:
UserTesting interview excerpts on sleep
- “I constantly cannot turn my brain off at night. I do not get the sleep that I am required, uh, seven hours, eight hours per night. That is a pipe dream. I’m lucky to get two and a half, three hours per night. I’m just consumed with daily stressors and my mind never turns off. Um, very uncomfortable throughout the day trying to work through sleeplessness. So a hundred percent, I’d give this an eight out of seven.”
- “I operate best anywhere between six and eight hours of sleep, and I feel that I typically will get that on a normal basis unless I have some big issue happening.”
- “Um, going to bed at 11 and waking up at five or 6:00 AM is really, really hard. Um, and so yes, it’s exhausting. And so trying to get out the door in time to get to where I need to be does affect my health and my sleep.”
- “Nah, I get plenty of sleep. Um, you know, I usually go to bed around 11. I get up around six. That’s plenty of seven hours of plenty of sleep for me.”
- “I work long hours. Uh, I deal with my kids and I get home late and I go to bed like 10 L chunk, get everybody to bed. So it’s kind of frustrating. It’s very, very hard to sleep.”
- “Um, well, I work a lot. Um, I have other things to do. I have to get up early, I have to get back home, do other, do things that I have to do. And then, um, yeah, so because of that, uh, because I have a lot of things to do, um, I don’t get enough, I don’t get enough sleep. I think you can actually see that I, I’m, I’m, I’m actually already tired at the moment, so, uh, definitely I, I don’t get enough sleep.”
Synthetic Users also made up some bizarre characteristics for its participants. Each of my six “participants” used a different form of transit: carpool, car, bicycle, bus, train, and subway. Who rides a bike for more than an hour each way to and from work? I’m sure someone in America does, but you wouldn’t find them in a random sample of six people. The UserTesting participants were more like typical people: five of them drive, one uses a mix of car and public transit.
Quality of insights: a big difference. To me, the responses from Synthetic Users seemed extremely reasonable, but also unrealistically self-aware. I thought they read a bit like the recommendations you’d get from a self-help book. For an example, see the quotes above about creating a bedtime routine and sleep schedule. Those sound like great things to do, but in the real world people are rarely so systematic and well organized.
Why do we do discovery research in the first place? When I worked at Apple they taught us not to focus on the things customers ask for, but new ideas that could delight them if we made them possible. Discovery research enables that ideation, by helping us develop intuition about customers’ thinking and reactions. The quirkiness, diversity of answers, and variety in phrasing that you get from human interviews feed your intuition. In contrast, the synthetic participants feel averaged out, like a stone sculpture that’s been sandblasted until you can only see its basic outline.
I’m sure an AI system can be configured to make its results sound more quirky, but since the system is all based on averages, I think it’ll be very hard to know if the quirks you’re seeing are genuine or random things hallucinated by the model. As a result, any intuition you create from those details will also be suspect. You’ll think you know your customers when you really don’t.
There was also a big difference between the platforms in numerical scoring. In both systems, participants were asked to rate the product idea on a 1-5 scale, five being best. There was a dramatic difference in the responses. As shown in the chart below, the Synthetic Users scores clustered tightly around 2.5, while the UserTesting ratings were more diverse and tended toward five. Because of the small sample size, you don’t want to read too much into any numerical result, but this sort of rating question is usually a strong directional indicator of the potential demand for a product. In the controlled atmosphere of an experience test, people usually overstate their willingness to buy something. So if you can’t get a lot of them to give it a five, you’re in trouble.
The UserTesting result gives a hint that there may be hope for a flying rideshare system. The Synthetic Users result, on the other hand, seems to indicate that even people with long commutes would be ambivalent about the product. If I saw those scores in a test of real people, I’d say the product was in deep trouble.
Followup questions: Interesting but sometimes prone to errors. An intriguing feature of the Synthetic Users system is that you can ask instant followup questions. Since the “users” are artificial, you can type in followup questions at any time, and the system will synthesize immediate answers. I tried some followup questions, and received fluent-sounding results. However, the information delivered by the system sometimes got muddled:
- A “user” who supposedly had three children claimed to have two in a followup question
- As an attempted trick, I asked a “user” who supposedly lived in Los Angeles why they lived in Chicago. The system failed to detect my trick: “I live in Chicago primarily because it’s where I grew up and where most of my family resides. It’s a city I know and love, despite the challenges of commuting and the fast-paced lifestyle. Additionally, my job is here and the city offers good educational opportunities for my children. It’s a balance of personal, professional, and family considerations.”
- A second trick, in which I asked a married “user” why they got divorced, was correctly detected: “I think there’s been a misunderstanding. I’m actually still married to my wife. We work together to manage our busy schedules and take care of our children. It’s challenging at times, but we’re committed to our family and to supporting each other.”
You can ask followup questions with the UserTesting system, but the process takes a lot more time (hours at least) and sometimes the participants may not respond at all.
The results summaries: Surprising similarities. Synthetic Users and UserTesting can both use AI to generate a summary of the test results. In Synthetic Users, the system summarizes the synthetic interviews it created. In UserTesting, the system summarizes the transcripts of the interviews.
There were some differences between the summaries, but I was surprised by the similarities. Here’s how Synthetic Users summarized what’s appealing and concerning about a flying rideshare service:
Synthetic Users summary of what’s appealing and concerning
Appealing:
- “Users appreciate the potential of the proposed solution to address their commute-related challenges and improve their work-life balance.
- They see the reduced commute time as a significant benefit that would provide them with more time for rest, family, and self-care.
- Users are intrigued by the convenience of having the aircraft pick them up and drop them off at their doorstep.
- They recognize the potential for stress reduction and improved well-being that could come from a shorter and more enjoyable commute.
- Users understand that the proposed solution may not comprehensively solve all of their problems, but they see it as a step in the right direction.”
Concerns:
- “Users have concerns about the safety and reliability of an AI-controlled aircraft and the potential risks or malfunctions associated with it.
- Affordability is a major concern, as users want to ensure that the cost of the service is reasonable and accessible for individuals with varying income levels.
- Users are concerned about the potential environmental impact of introducing more aircraft into the transportation system and the need for sustainability measures.
- The lack of control over work demands and the challenges of finding reliable and affordable childcare options are also concerns for users.”
Here’s how UserTesting summarized what’s appealing and concerning:
UserTesting summary of what’s appealing and concerning
Appealing:
- “Safety concerns are raised by multiple contributors, particularly regarding the AI-controlled aircraft without a pilot.
- Most contributors find the idea of a quick, stress-free commute appealing, with the potential to save time and multitask during the ride.
- Several contributors express concerns about the cost of the service and whether it would be affordable.
- Some contributors mention the possibility of selling their car or reducing the number of cars they own if they rely on this service.
- A few contributors appreciate the idea of not having to pay attention to the road and being able to focus on other tasks during the commute.
- 1 contributor highlights the potential health benefits of reduced stress from avoiding traffic and commuting.
- 1 contributor suggests more testing should be done before they would feel comfortable using the service.”
Concerns:
- “1 contributor emphasized the importance of maintenance and vetting of the flying apparatus to ensure safety.
- Several contributors mentioned concerns about the pricing and logistics of the service.
- Some contributors wondered about the service’s ability to accommodate emergencies or the need to leave early.
- Most contributors expressed concerns about the safety of the service.
- A few contributors had questions about the scheduling and availability of the service.
- 2 contributors mentioned concerns about the reliability of the AI system and its potential for crashing.
- 1 contributor was unsure about the cost effectiveness of the service compared to owning a car.”
The two platforms both identified customers’ main concerns: safety and price. You could say that this shows Synthetic Users is a good proxy for real discovery interviews, but on the other hand you don’t really need any research at all to guess that people worry about safety and price when flying. If you’re looking for non-obvious insights, the platforms had different results:
- Synthetic Users predicted that customers would have questions about environmental impact, an issue that did not come up in the UserTesting responses.
- UserTesting participants raised some other issues that did not come up in Synthetic Users:
- Could I get unscheduled transit in an emergency?
- Would this enable me to sell one of my cars?
- I might appreciate being able to do work during the commute
How does all this compare to ChatGPT?
Aa a final test, I was curious about how the results from Synthetic Users and UserTesting would compare to what I can get for free from a generative AI service. I logged into a free ChatGPT 3.5 account and input the following query:
Query to ChatGPT
There is a new rideshare service in which an aircraft lands at your house, picks you up, and takes you to your office. The ride takes no more than 20 minutes door to door. There is no pilot in the aircraft; it is controlled by an artificial intelligence. You can return home the same way.
What about this service, if anything, would be appealing to customers, and why? Would customers view it as improving their lives?
What concerns would customers have about this service?
On a 5-point rating scale, with 1 meaning not at all appealing and 5 meaning extremely appealing, how would an average customer rate the appeal of this service to them?
Describe the demographics of a typical customer for this service. How often do they commute to work? How long is their commute? Are they likely to have school-age children at home? What other demographics make them distinct from the average person?
Here’s the response I received in a few seconds:
ChatGPT 3.5 response
The concept of a rideshare service using AI-controlled aircraft for a quick and efficient commute presents several potential appealing aspects for customers:
- Time Efficiency: The promise of a door-to-door commute in just 20 minutes is highly appealing. This service could significantly reduce commute times compared to traditional ground transportation.
- Convenience: The convenience of being picked up and dropped off at one’s doorstep without the need for a pilot or additional stops could be a major selling point.
- Productivity: Customers might appreciate the potential for increased productivity during their commute, as they could use the time for work or leisure activities instead of driving.
- Novelty and Innovation: The use of AI-controlled aircraft adds a futuristic and innovative element, attracting customers who value cutting-edge technology.
- Reduced Traffic Congestion: As the service involves flying, it could potentially alleviate ground traffic congestion, making the commute smoother.
Concerns customers might have about this service include:
- Safety: The absence of a human pilot might raise concerns about the safety and reliability of the AI-controlled aircraft.
- Regulatory Issues: Customers may worry about the regulatory framework and safety standards for such autonomous aerial vehicles.
- Environmental Impact: The environmental impact of flying vehicles, including noise pollution and emissions, could be a concern.
- Cost: The cost of such a service might be a factor, and customers may compare it to existing transportation options.
- Accessibility: Not everyone may have the infrastructure or space for aircraft landings and takeoffs near their residences.
On a 5-point rating scale, the appeal of this service would likely vary among individuals, but it could be moderately high, with many customers likely rating it between 3 and 4 due to the time savings and convenience factors.
The demographics of a typical customer for this service might include:
- Professionals: Those with demanding jobs who value time and productivity during their commutes.
- Urban Dwellers: People residing in urban areas with high traffic congestion.
- Tech Enthusiasts: Individuals who embrace and enjoy cutting-edge technology and innovations.
- High-Income Earners: The service may attract individuals with higher disposable incomes willing to pay for premium transportation.
- Frequent Commuters: Individuals who have daily commutes, especially those with longer commutes.
- No School-Age Children: The service might be more appealing to individuals without school-age children, as family dynamics and needs could influence the choice of transportation.
It’s important to note that customer demographics can vary, and the success of such a service would depend on addressing both the appealing aspects and potential concerns mentioned above.
You should judge for yourself, but to me, the issues and ideas I got for free from ChatGPT seemed very similar to what I got from Synthetic Users. ChatGPT also flagged some issues that came up in real interviews but not in Synthetic Users (for example, customers might enjoy using the flying commute time to do other tasks). Chat GPT also predicted some things that I didn’t expect (for example, core customers would be people without kids). I’d want to test those through real research.
Overall, I think I learned about as much through a free ChatGPT query as I did through the Synthetic Users process. I didn’t get the detailed simulated interviews that Synthetic Users produces, but in terms of ideas, the results were about the same.
What it all means
Is generative AI in its current form a good substitute for discovery interviews? Based on this test, I think the answer is no:
- Synthetic interviews put you at risk of creating participants who don’t exist in the real world, reinforcing any mistaken assumptions you have made about the market. Real research forces you to confront customer realities.
- Synthetic participants reflect the average across all the data the system has been fed. You don’t get a good read for the diversity of real customers, and this makes it hard to create insights about how real people feel and think.
- The absence of videos makes it hard to understand the emotions of customers and empathize with them.
- Some nuances of customer opinion are not predicted by the AI system.
Synthetic Users and ChatGPT are definitely much faster than traditional research, but that gap is narrowing. Old school in-person interviews typically take weeks to recruit and conduct. That timeline has already been cut to days through online services and panels. Self-interviews paired with AI-generated summaries are making another big cut in turnaround time, by freeing researchers from the need to participate live in every moment of the interviews.
Although I don’t think generative AI in its current form is a good replacement for discovery research, I think it is a good supplement to the process. I would be comfortable using it to brainstorm issues to explore in research. For example, how many people worry about the environmental impact of a flying car? That’s a good question that I hadn’t thought to ask on my own.
I think generative AI is also useful for creating summaries of interview data. I was very comfortable with the quality of the AI-generated summaries created by all three platforms.
Ironically, I think the most problematic element of Synthetic Users is its headline feature: the synthetic interviews themselves. In attempting to create convincing fake interviews, the system introduces hidden inaccuracies that could easily lead a product team in the wrong direction. I think it’s better to treat generative AI as something new, with its own strengths and weaknesses — and to focus on the things it does uniquely well — rather than making it pretend to be something it isn’t.
If you want to do your own comparisons, here’s the source information:
- Summary and interview text generated by Synthetic Users
- Test plan from the UserTesting study
- AI-generated interview summaries created by the UserTesting platform
- Raw interview transcripts from the UserTesting study
Thanks to Ranjitha Kumar, Kevin Legere, and Tom Hayes for providing feedback on drafts of this article.
Image generated by Dall-E 2.
The opinions expressed in this publication are those of the authors. They do not necessarily reflect the opinions or views of UserTesting or its affiliates.